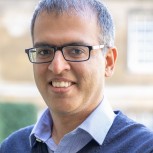
Professor of Information Engineering
Academic Division: Information Engineering
Research group: Signal Processing and Communications
Telephone: +44 1223 7 66767
Email: rv285@eng.cam.ac.uk
Research interests
My research interests include information theory, high-dimensional statistics, statistical machine learning, and signal processing. A detailed description of my research can be found here.
Teaching activity
Current teaching:
- 3F7 Information Theory and Coding
- 2P6 Communications
Research opportunities
Other positions
Staff Fellow, Trinity Hall
Biography
I am Professor of Information Engineering at the University of Cambridge and a Staff Fellow at Trinity Hall. I am part of the Signal Processing and Communications Lab in the Division of Information Engineering.
I received my PhD in Electrical Engineering (Systems) from the University of Michigan, Ann Arbor in 2008, and my undergraduate degree from the Indian Institute of Technology, Madras in 2002. Before joining the University of Cambridge in 2013, I held post-doctoral positions at Stanford University and Yale University.
My research interests include information theory, high-dimensional statistics, signal processing, and machine learning. I am an Associate Editor of the IEEE Transactions on Information Theory, and from 2018-21 was a Fellow at the Alan Turing Insitute, and an Associate Editor of the IEEE Transactions on Communications.