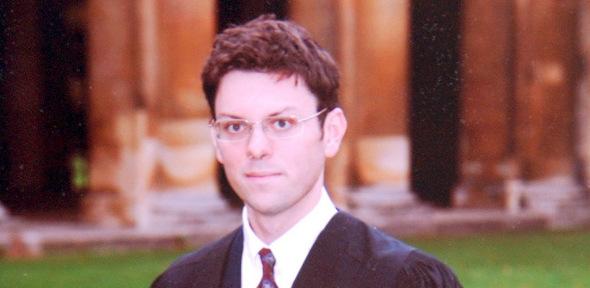
Congratulations to Andrew Wilson for winning the Best Student Paper Award at the 2011 Uncertainty in Artificial Intelligence Conference.
Andrew Wilson is in his second year of a PhD in machine learning, in the Computational and Biological Learning Group. Machine learning is partly inspired by advances in neuroscience, and is focused on developing algorithms for learning and decision making. Andrew entered the field with a background in physics and mathematics, and as a classically trained pianist who especially enjoys Bach and Glenn Gould. He decided to focus on machine learning because it is a young field which is quickly growing and changing, with many new ideas, and wide ranging applications in physics, music theory, economics, medicine, and in general, statistical analysis of complicated data.
Andrew's award winning paper, co-authored with Zoubin Ghahramani, develops a new family of random processes, "Generalised Wishart Processes", for time and spatially varying uncertainties and correlations. We can imagine measuring the position of a rocket as an example of changing uncertainty: the further away the rocket gets, the more unsure we are about where it is. We can also imagine changing correlations. If there is general enthusiasm about technology companies, the price of Microsoft and Apple shares may increase together. On the other hand, if customers are purchasing Microsoft products instead of Apple products, Microsoft shares may increase while Apple shares decrease. Such relationships will change over time.
Modelling uncertainty and correlations is fundamental in statistics; however, one often assumes that these uncertainties and correlations are fixed, and this assumption can have a significant impact on statistical inferences. With a Generalised Wishart Process, we no longer need to make this assumption, because we can learn how uncertainties and correlations change over time and space. This process is presently being used to model how correlations between neuron firings vary over time, for a better understanding of how the brain works. It is also being applied to predict changing correlations between greenhouse gases and solar radiation as a function of time and geographical location, and to forecast uncertainties and correlations for high frequency financial assets. Previously these applications were inaccessible. Generalised Wishart Processes are particularly promising because they can naturally handle high dimensional data sets, like a large portfolio of stocks instead of just two or three, and different types of correlation structures, like correlations that change periodically with time.