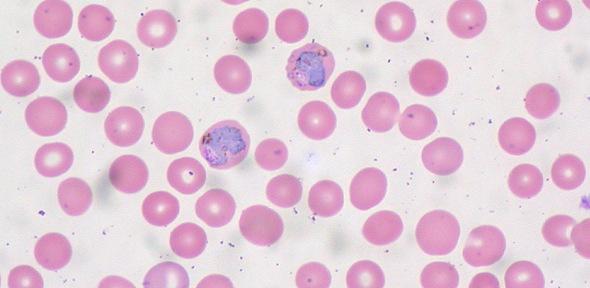
More than one million people die from malaria every year - most of them in the world's poorest areas. While it's no surprise to find Cambridge scholars at the forefront of the battle against the disease, crucial research is underway not just in subjects like biochemistry and physiology, but in the unlikely setting of the Department of Engineering.
New mathematical questions are being generated all the time as more comparative gene finding gets underway. By answering them, we may ultimately be able to help to solve a whole range of biological problems.
Dr Karsten Borgwardt
Malaria is one of the world's worst health problems and one of its biggest killers. We may not feel its impact very often here in the UK, but in South and Central America, Asia, the Middle East and - in particular - Africa, it can be devastating.
According to the World Health Organisation, more than 1 million people die from its effects every 12 months. Children in Africa can expect to have between one and five episodes of malaria fever in a year. And every 30 seconds, malaria kills another child.
The sheer scale of that impact has led to internationally-backed initiatives such as Roll Back Malaria, which aims to have significantly reduced global deaths from the disease by 2010. Treating it, however, and creating the as-yet-elusive malaria vaccine, is easier said than done. To cure it, you have to know how it works. To do that, you have to understand what makes malaria parasites similar to or different from one another. And to achieve that, you have to know where to look.
Which takes us from the world's worst malaria hotspots to the unlikely setting of the Department of Engineering. Strange as it may seem, researchers here are producing computer software which has already proven essential in the global fight against the disease.
The work is being undertaken in the Department's Machine Learning Group. This team of researchers, led by Professor Zoubin Ghahramani, focuses on understanding and devising computer algorithms - the sequences of operations which allow computer programmes to perform a given task. It was precisely one such set of algorithms developed by Dr Karsten Borgwardt which towards the end of last year helped scientists at the Sanger Institute near Cambridge to decode the genetic make-up of the malaria parasite Plasmodium knowlesi.
Until recently, only four species of malaria were known to affect humans. Plasmodium knowlesi was thought mainly to be a cause of malaria in monkeys, but in fact scientists had been grossly underestimating its effects. Today, it is emerging as the fifth human malaria parasite and is particularly dangerous in south-east Asia, where people often live close to the monkeys and mosquitos which carry it.
Although all malaria parasites are similar, each new species has a unique set of tricks and disguises which stop the host's immune system from fighting it off. This is all the more important because to treat malaria rapid recognition and an early response is vital. By decoding its genome - the complete set of genetic information controlling its behaviour - scientists were able to spot the "cloaking devices" used by Plasmodium knowlesi and move a step closer to developing drugs and vaccines to fight the disease.
But what does all this have to do with algorithms developed at the Engineering Department? To crack Plasmodium knowlesi's code, scientists used a technique called "comparative gene finding". This involves taking a similar species (another malaria parasite) whose genes have already been located and identified, and then looking for the same genes in the unmapped species.
"In any genome we have a very long string of sequence information," Karsten explains over the phone from the Max Planck Institute in Tübingen, Germany, where he now works. "To work out the position of the different genes within the genome we need to find parts of the sequence which are shared by the genome we already understand."
The problem is where to look. Any genome contains a baffling quantity of sequence information which makes spotting the similarities and differences rather like trying to find a needle in a haystack. Fortunately, this is precisely what computer algorithms are designed to do: The software developed by machine learners allows computers to extract useful patterns, rules and information from a huge jumble of data.
In this case, Karsten, working closely with Professor Irmtraud Meyer at the University of British Columbia in Canada as well as Dr Arnab Pain and other researchers at the Sanger Institute, developed software which enabled scientists to make predictions about where they might find certain genes in the sequence.
"Imagine it this way," Karsten says: "We want to be able to give a label to every part of the genome sequence as we move along it. We want to be able to say: 'This part of the sequence affects that gene, this part of the sequence belongs to this part of this gene."
"Finding the most likely label for each part of the sequence is basically a mathematical problem - it depends on probability. Machine learning and statistics can help scientists to label the sequence and establish how the parasite works. Because not every parasite is the same, they can then spot the genes which are only present in one particular malaria species."
Ultimately, it is this knowledge of the parasite's code that will lead scientists to creating a vaccine. In the meantime, Borgwardt and his colleagues are interested in how they might provide similar support to those mapping the genomes of entirely different species using the same comparative method.
"The probability of finding a gene that is similar to a gene of a related species depends on which species you are looking at," he adds. "This means that our algorithm has to be able to adapt to each genome you want to decipher. New mathematical questions are being generated all the time as more comparative gene finding gets underway. By answering them, we may ultimately be able to help to solve a whole range of biological problems."
The Machine Learning Group, here at the Department, are working on a number of research projects at the intersection of machine learning and computational biology, ranging from new algorithms for predicting how proteins fold, models for learning the regulatory networks of genes, to methods for discovering biomarkers for diseases.
This article originally appeared in 'Newsletter' The Magazine for the Staff of the University of Cambridge, February/March 2009.