
Cambridge PhD student Danielle Saunders is studying machine translation systems with the aim of reducing instances of gender bias by “fine-tuning”, rather than retraining the language output. It forms a growing area of research which poses wider questions to do with gender stereotyping in society.
I don't think reducing gender bias in translation alone will break stereotypes, but I hope that working on the problem will make people more aware of it, and of the stereotypes that we can make or break with language use in our lives.
Danielle Saunders
This approach is believed to be the first attempt of its kind in Neural Machine Translation (NMT). NMT uses an artificial neural network (based on the human brain) to predict the likelihood of a sequence of words to automatically translate from one language to another. It is the quality and accuracy of these translations however, in relation to instances of gender bias, which has been the subject of a recent paper co-authored by Danielle and Professor of Information Engineering, Bill Byrne. The paper is due to appear at the upcoming Annual Conference of the Association for Computational Linguistics in July.
Titled Reducing Gender Bias in Neural Machine Translation as a Domain Adaptation Problem, the paper aims to minimise the impact of gender bias on translation quality by exploring the issue at sentence-level. For example, faced with the sentence, “The doctor told the nurse that she had been busy,” a human translator would try to understand its meaning and would infer that ‘she’ refers to the doctor, before correctly translating the word in German as Die Ärztin. “But machine translation systems don't understand language in the same way”, says Danielle. “The model only knows that for certain English words, like ‘the doctor’, the German translation usually contains the words ‘Der Arzt’ – the masculine form. This is because in the biased data it has seen, most doctors are male. So in an uncertain case like this, it defaults to the form that it has seen most frequently.”
The researchers demonstrate their approach by translating “tiny, handcrafted gender-balanced datasets” from English, into German, Spanish and Hebrew – languages chosen for their varied linguistic properties. The researchers also hope to demonstrate that gender bias can be removed in the language output of a number of commercial machine translation systems: Google, Amazon, Microsoft, and SYSTRAN.
“Gender bias in machine translation comes from bias in training data, for example, news reports, political speeches, etc. It reflects the society that produces it, where a nurse is less likely to be a man and an engineer is less likely to be a woman,” said Danielle. “I don't think reducing gender bias in translation alone will break those stereotypes, but I hope that working on the problem will make people more aware of it, and of the stereotypes that we can make or break with language use in our lives.”
Reference:
Saunders, D., Byrne, B. Reducing Gender Bias in Neural Machine Translation as a Domain Adaptation Problem. ACL 2020. arXiv:2004.04498
About Danielle Saunders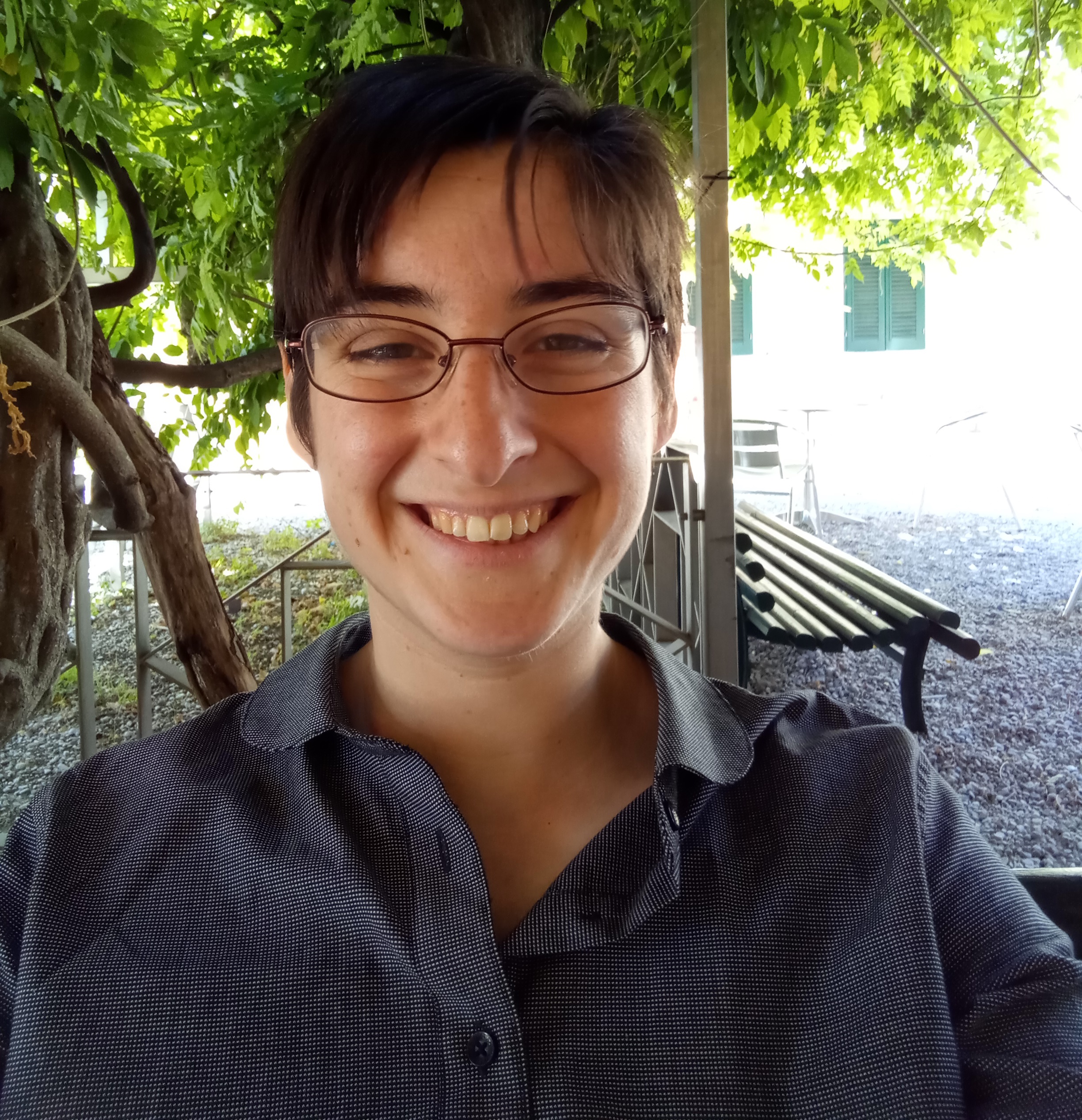
Danielle is part of the Department’s Machine Intelligence Laboratory. Her PhD research project is in Statistical and Neural Machine Translation, focusing on domain adaptation. Recent investigations have included: gender debiasing, domain adaptation to unseen text at test time, adapting models to biomedical translation, and use of syntactic annotation.
Here, she explains more:
I find machine translation fascinating generally. It's a very hard problem, but one where there has been huge progress in the last few years. I'm particularly interested in domain adaptation, which is adapting machine translation systems to language it might not have seen before, such as scientific papers or legal documents. So when I saw a presentation about the gender bias problem in machine translation, I wondered whether you could adapt a model to language without gender bias in the same way. That then led to this work.
I would definitely recommend other women to pursue careers in artificial intelligence (AI) and machine translation. The gender balance is skewed, but not as much as I was expecting when I started my PhD, and every year I see more women getting involved, whether that's with masters projects or in industry. It's an area where people are developing and applying new technologies incredibly fast, and that means there are always interesting new problems to work on.