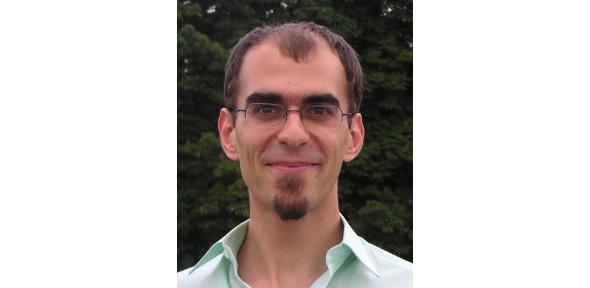
Congratulations go to the Department's Sébastien Bratières who is a Yahoo! 2010 Key Scientific Challenge Winner, one of 23 Students, from 16 world-class Universities. The winners were selected based on their research proposals into the 12 different scientific challenges they believe are critical to fueling innovation on the Web.
The Internet is 27 years old, give or take a few years and depending on whom you're asking. But while the Web has changed a lot in our lives the last couple of decades, by historical standards the science of the Internet is still relatively young.
It is cliché to compare the Internet to Gutenberg's invention of the printing press, but we forget that it took more than 200 years after the invention of the printing press until we experienced the rise of the novel as a popular art form. In other words, the Web we have now is not the Web we'll have in the future. There's a tremendous amount of innovation to come. The question is: "what kind of innovation can we expect?"
Yahoo! Labs believe that innovation in the Web experience of tomorrow will depend directly on the work being done behind the scenes today to create new scientific theories, models and disciplines for understanding the Internet today. In fact, a core element of Yahoo! Labs' charter is to invent the new sciences that will underlie the next generation Internet. That charter is evident in their Key Scientific Challenges Program, which focuses on supporting the bright young minds at universities across the world who are thinking, researching and creating those new sciences.
Each of the winning students submitted to Yahoo! an idea and a research proposal that Yahoo!'s scientists and leaders saw as a genuine contribution to their field and to an area seen as critical to laying that scientific foundation for innovation in the future experience of the Web.
The practical significance of Sébastien's proposed work is as follows. Society is overwhelmed with data, this is true for individuals (e.g. emails, social networking sites, news), for companies (transactions, clients, employee records), for governments (census data, medical records, immigration, crime reports), and for scientists (weather and climate data, genomic data, astronomical data, pharmaceutical data). To cope with and benefit from such vast amounts of data, we need methods to be able to model the data, predict from the data, filter the relevant from the irrelevant, organise, and visualise the data. Machine learning methods provide a method for solving these important problems. Probabilistic (Bayesian) machine learning methods are necessary to handle the underlying uncertainties, noise, incompleteness, and heterogeneity of real-world data sources. Unfortunately, probabilistic machine learning methods become computationally too demanding when applied to the vast data sets of societal and scientific significance. It is therefore of critical importance to develop parallel and distributed approaches to efficient data modelling so that these computations can be distributed on large “farms” of distributed computer servers, so that society can benefit from the myriad applications of machine learning methods. It is this critical research problem which Sebastien is trying to address in his PhD.
Sébastien's project brings together two strands of research that receive a lot of discussion these days:
The first is Bayesian probabilistic modelling, abstractly speaking, this is to create mathematical models (ie representations) from observed data. These models can be used to "simulate" the process which gave rise to the data in the first place, even if very crudely. Moreover, it does so while mimicking the frequency distribution of the observed data. This in turn is operational for a range of data-related tasks: classifying, labelling, clustering, prediction, guessing missing values. Examples of such models are: representing how the part-of-speech of a word depends on the parts-of-speech of its neighbouring words in sentences, and therefore automatically labelling words with their part-of-speech (useful for further language processing); modelling how customers respond to ads displayed on websites, according to keywords on the page, and other context information about the user, her navigation; modelling viewers' tastes for movies, according to the movie's attributes, their own profile, other movies they have liked, this allows for recommendation, or catalogue analysis/construction; modelling the spatial distribution of ores in the earth crust, etc. The idea is always that some data is available, and that we want to learn from it to carry out one of the mentioned tasks.
The issue with Bayesian modelling is that it often involves summations (such as integrals) of functions over very high-dimensional spaces, with millions of dimensions. An example is a greytone image of 100x100 pixels is determined by 100x100=10,000 pieces of data, each of which can vary along one dimension, so 10,000 dimensions. To cope with these huge summations, massive computational power is needed. So far, parallel computing has been popular: it runs several processors on one PC, which share the PC's memory and each tackle part of the task at hand before merging their results. Parallel computing goes only so far as the computation can be carried out on a single PC.
The other strand is concerned with overcoming this limitation: it involves distributed computing, where several (10 or 100 or 1000) PC's share a task and merge their results. This is very popular because of a background trend in the IT industry to sell computing services, ie central processing units (the portion of a computer system that carries out the instructions of a computer program), power by the hour. Related IT buzzwords are cloud computing and virtualization. Sebastien's project consists of exploiting distributed (cloud) computing architectures to carry out the computation involved in applying Bayesian learning to web-scale datasets.
If you are interested in learning more about the other key challenges or are curious about why Yahoo! have chosen these specific scientific challenge areas, some researchers shared their opinions on what makes these problems important in the Key Scientific Challenge series on Yodel http://ycorpblog.com/ the Yahoo blog site, with posts on Green Computing, Privacy and Security, Economics and Social Systems, Advertising, Web Information Management, and Machine Learning.
The 2010 winners, in addition to receiving US$5,000 in unrestricted seed funding, will convene at Yahoo! headquarters in Sunnyvale, California for the exclusive Key Scientific Challenges Graduate Student Summit where they will spend two days with the Yahoo! Labs scientists presenting their work and jointly discussing the future of these fundamental scientific challenges and ultimately how their research can have the greatest impact on the next generation of the Internet.
Sébastien has also received an extra US$4000 from Amazon Web Services to carry out computations on the Amazon Computing Cloud, Amazon's product for "computing as a service". This ties in with the project he received the Yahoo award for, in that it provides him with concrete means to carry out the computations needed for this research.