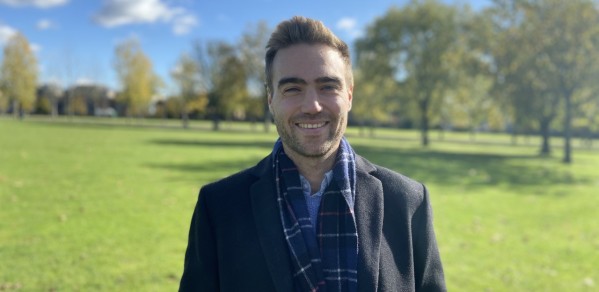
PhD student Joshua Nevin has been accepted into the Turing Enrichment Scheme for 2021 at the Alan Turing Institute.
I believe that utilising our knowledge of the system physics and explaining the predictions of algorithms is crucial for the deployment of machine learning approaches in real-world engineering problems. This allows us to build confidence in machine learning amongst operators and users of data-driven systems, as well as helping algorithms to work successfully in situations where the availability of data is constrained.
Joshua Nevin
Joshua Nevin is in Professor Seb Savory’s group, the Fibre Optic Communication Systems (FOCS) Lab in the Department of Engineering, Electrical Engineering division.
Josh summarises his research and plans for the enrichment placement: "My research broadly encompasses explainable machine learning approaches for the design and operation of optical fibre communication systems. Specifically, my interests include the design and application of physics-informed machine learning methods, the intersection of explainable machine learning and reinforcement learning, approaches for digital twins in optical networks and the design of machine learning algorithms for situations where data availability is strongly constrained.
"I am currently investigating how Gaussian processes can be used to estimate the performance of deployed optical network components from a constrained volume of monitoring data, for applications in digital twins.
"During the enrichment placement I plan to utilise the world-class expertise that the Turing has to offer, particularly in the data-centric programme. I am looking forward to engaging in new collaborations and delivering explainable data-driven methods that leverage knowledge of system physics.
“I believe that utilising our knowledge of the system physics and explaining the predictions of algorithms is crucial for the deployment of machine learning approaches in real-world engineering problems. This allows us to build confidence in machine learning amongst operators and users of data-driven systems, as well as helping algorithms to work successfully in situations where the availability of data is constrained."