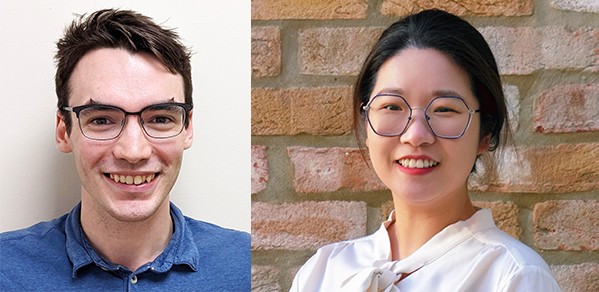
Cambridge engineers exploring how innovative applications of AI can be deployed in research and real-world contexts, have been awarded funding in recognition of tools capable of delivering benefits for science and society.
Our ongoing research is showing the potential for machine learning and imaging to improve care in NICUs. The funding will allow us to start conceptualising a device that can put our research into clinical practice.
Dr Alex Grafton
The 2023 Accelerate Science and Cambridge Centre for Data Driven Discovery (C2D3) funding call has supported 11 projects including two from the Department of Engineering. They are:
- Meerkat neonatal monitoring – embedded device development.
- Embodied AI and bio-inspired soft robotics – a workshop bringing together experts from AI, robotics, bioengineering and related fields.
Meerkat: a second set of eyes for nurses
Dr Alex Grafton is a Research Associate in Non-Contact Neonatal Monitoring. Working in collaboration with the Department of Paediatrics, Dr Grafton is harnessing the power of machine learning technology and computer vision to provide a second set of eyes for nurses working in Neonatal Intensive Care Units (NICU).
Specialised care for premature and sick newborn babies is required around-the-clock from a team of experts. With so much pressure on nurses’ time, Dr Grafton envisages that Meerkat could provide continuous monitoring of each baby, ensuring that both acute clinical events such as seizures, and long-term changes in behaviour such as a baby becoming lethargic, can be noted, and acted upon.
Machine learning allows the researchers to extract, via a computer, information from video footage collected of each baby. This means that interventions such as when staff or a parent’s hands are in the cot, can be detected in real time, along with the ability to automatically locate the baby in the video frame and monitor the baby's behaviour.
The project will use real-world data to optimise machine learning models for edge computing on embedded devices in the NICU environment. Edge computing involves moving the computation closer to the data source so that data, in this instance, can be processed in the NICU, cot-side if possible. Keeping data on-site is also an important requirement for patient privacy.
An example of what Meerkat "sees" in the incubator. Credit: Dr Alex Grafton.
“Our ongoing research is showing the potential for machine learning and imaging to improve care in NICUs,” said Dr Grafton. “The funding from Accelerate Science and C2D3 will allow us to start conceptualising a device that can put our research into clinical practice.”
The collaboration with the Department of Paediatrics began during Dr Grafton’s PhD five years ago, when he was investigating how 3D cameras could be used for monitoring respiratory function in adults and children.
“The idea for Meerkat came about through an existing link between the Departments of Engineering and Paediatrics and we saw opportunities for how the imaging equipment could be used to support neonatal care.
“Nurses are very busy preparing feeds, medicines, changing nappies and keeping records, all while caring for more than one baby. This means that they can't continuously watch each baby, so this is where we envisage Meerkat will provide a second set of eyes.
“We have collected a large amount of video data already in our proof-of-concept study, which we can use to train machine learning models on what sort of movements and behaviour it should be detecting. Providing continuous monitoring of this sort would not be possible without machine learning.”
Dr Grafton added: “There is also a lot of research globally that involves monitoring vital signs using cameras, so we are also looking at how the 3D camera can be used to measure heart rate and respiratory rate, without the need for contact with the baby. Our video could also be saved for analysis by child physiotherapists, who would then be able to examine a baby's movement to assess development.”
Workshop: Embodied AI and Evolutionary Soft Robotics
Diagram illustrating the optimisation framework to generate diverse gripper designs for soft robots. Credit: Dr Yue Xie.
Dr Yue Xie is a Marie Skłodowska-Curie Future Roads Fellow and a Research Associate in the Bio-Inspired Robotics Laboratory (BIRL). Dr Xie is part of a project team that will host a workshop on Wednesday 6 March to gain a comprehensive understanding of the challenges and opportunities presented by AI in robotics.
During the workshop, Dr Xie will present her latest research findings on embodied AI and soft robotics. Her research is centred on the application of bio-inspired evolutionary computation, for example, genetic algorithm, ant colony algorithm, particle swarm optimisation and so on, particularly in embodied AI, soft robotics, and intelligent traffic management.
Her focus is on integrating these computational methods into designing robotic morphology and control strategies to enhance their adaptability and efficiency and develop approaches to optimise traffic systems. With autonomous vehicles expected to have a significant impact on future roads, Dr Xie’s research will integrate multi-agent system methods and information theory for enhanced coordination and efficiency.
Dr Xie said: “Being a recipient of this funding is both an honour and a significant responsibility. Personally, it serves as a validation of the hard work and dedication that I have invested in my research. It reinforces my belief in the potential of bio-inspired evolutionary computation and its transformative impact on fields like embodied AI and soft robotics.
“Professionally, this funding is crucial support, enabling me to push the boundaries of my research further. It allows me to communicate with leading experts and provides more opportunities for me as an early-stage researcher.”
Adapted from an Accelerate Science news article.