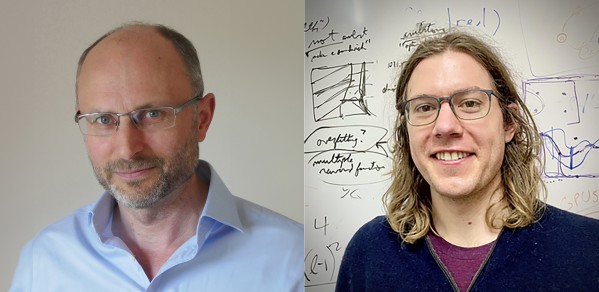
Professor Matthew Juniper and Dr David Krueger have been appointed fellows of the Alan Turing Institute. Turing Fellows are the next generation of world leading researchers. They have proven research excellence in data science, artificial intelligence, or a related field.
I’m delighted to welcome a new cohort of Turing Fellows, brought to us from across our University Network in recognition of their status as the next generation of world leading researchers in the data sciences, AI and related fields.
Professor Mark Girolami, Chief Scientist, The Alan Turing Institute
The Turing Fellowship Scheme aims to grow the data science and AI ecosystem in the UK by supporting, retaining and developing the careers of the fellows.
Professor Mark Girolami, Chief Scientist, The Alan Turing Institute said:
“I’m delighted to welcome a new cohort of Turing Fellows, brought to us from across our university network in recognition of their status as the next generation of world leading researchers in the data sciences, AI and related fields.
“I’m very much looking forward to seeing the immense value they will add to our diverse and vibrant science and innovation community, including playing a critical role in the delivery of the Turing strategy as we strive to change the world for the better through data science and AI.”
Professor Matthew Juniper
Matthew Juniper's research is at the interface between physics-based learning and machine learning.
Matthew said: "John von Neumann, one of the 20th century's most outstanding mathematicians, is credited with saying 'with four parameters I can fit an elephant, and with five I can make him wiggle his trunk.' The implication, which remains influential today, is that physical models must contain few parameters. In the 21st century, many data scientists assert that models work just as well if they contain millions of parameters and no physics at all. My research started by wondering what von Neumann would say about this and then moved on to how 21st century physicists and engineers should respond."
Matthew's fellowship sits within the 'Fundamental AI' priority theme at the Turing Institute, which aims to advance the models, techniques, and principles that underpin AI. As well as being appointed a fellow, he has been seconded to the Turing Institute for research on Programmable Inference.
Matthew added: "David Mackay, Regius Professor of Engineering at Cambridge until 2016, is best known for his influential book 'Sustainable Energy without the hot air'. His main research, however, was on Information Theory, Inference, and Learning Algorithms. His work inspired me to view physics-based models as prior knowledge, their parameters as probability distributions, and data as the magic dust that reduces uncertainty. Fortunately, my primary research area turns out to be the missing tool that accelerates Mackay's methods sufficiently to tackle large problems. This means that these methods can now be used more widely in science and engineering."
The main thrust of Matthew's research is that physics-based models and neural network models are fundamentally similar in that they have some pre-defined structure and contain unknown parameters. These models extract information from data by filtering the data through this structure. The difference is that, in physics-based models, high quality prior information such as conservation of mass can be hard-wired, lower quality prior information such as physical parameters can be soft-wired as probability distributions, and data can be assigned some certainty relative to this prior knowledge. This systematic combination of physical prior knowledge and data allows information to be extracted more efficiently than can be achieved with a physics-agnostic neural network.
Matthew said: "I am amazed by the ability of humans to extract information from data. An AI algorithm might excel at a game by playing millions of times, while a human can be nearly as good by playing a few thousand times. How do we do this? I think we are projecting our observations onto an internal model of how the world behaves and then extrapolating to new imagined situations. With Programmable Inference, we can do this systematically, repeatably, and by respecting physical constraints. With adjoint acceleration, this becomes rapid and multi-dimensional. This is the essence of what I propose to develop with the Turing Institute over the next few years".
The first engineering application of this in Matthew's group is medical Flow-MRI, which is used to visualise blood flow through the heart and major arteries. In the laboratory, adjoint-acclerated Programmable Inference reduces scan times by a factor of 100 for the same quality of information.
"There is a long way to go before clinical application, but the promise is clear. If a 90 minute heart flow scan could be reduced to 60 seconds, such scans could be widely used to help monitor, prevent, and treat cardiovascular disease. Given that cardiovascular disease currently causes one quarter of deaths in the UK, this could have huge positive societal impact."
Dr David Krueger
David Krueger is an Assistant Professor in Machine Learning and Computer Vision. His work focuses on reducing the risk of human extinction from artificial intelligence (AI x-risk) through technical research as well as education, outreach, governance, and advocacy. His research spans many areas of Deep Learning, AI Alignment, AI Safety, and AI Ethics, including alignment failure modes, algorithmic manipulation, interpretability, robustness, and understanding how AI systems learn and generalize. He has been featured in media outlets including ITV's Good Morning Britain, Al Jazeera's Inside Story, France 24, New Scientist, and the Associated Press. David completed his graduate studies at the University of Montreal and Mila, working with Yoshua Bengio, Roland Memisevic, and Aaron Courville, and he is a research affiliate of Mila, UC Berkeley's Center for Human-Compatible AI (CHAI), and the Center for the Study of Existential Risk (CSER) at the University of Cambridge.
David said: "My research aims to clarify the risks of AI, and in particular societal and existential risks. I also develop and evaluate potential mitigations, such as alignment techniques.
"My work also informs approaches to governing AI. AI safety, like climate change, is a common good.
"The risks and externalities of AI systems are likely to affect all of humanity, and so we need to work together to address them through robust international policy responses.
"This is a grand socio-technical challenge, and my work highlights ways in which current approaches fall short and could be improved.
"Given the rapid rate of progress in AI, the lack of reliable methods for understanding and controlling AI systems, and the growing competitive pressure to adopt AI in many high-stakes applications, I believe we urgently need to prepare to coordinate to forgoe future AI technologies to a significant extent for a long time.
"I hope to motivate policymakers, AI researchers, civil society, and the broader public to accelerate efforts to address the risks of AI. I believe we are approaching scientific consensus that AI poses an existential risk to humanity. This was achieved for climate change several decades ago. We need to do better this time, given the rate of progress in AI, with many leading developers and researchers forecasting superhuman AI within 1-2 decades or less."
Turing Institute Fellowships
The new Turing Fellowship model is aimed at established researchers whose research interests align with the Turing’s Science and Innovation priorities outlined in the Institute Strategy.
As well as taking part in the Turing’s interdisciplinary, and collaborative research community, the new fellows will also support work in public engagement.
Research interests of the new fellows span everything from evolutionary studies, human genetics, energy justice and the future of cities to biodiversity loss.
The Turing Fellows were appointed through an open call which is anticipated to run on an annual basis. Future calls will be aligned closely to the Turing Institute’s goals.